Project title: From “tumblr purge” to “female nipples”: Telling a story of platform censorship critique through memes and digital methods
Lead: Elena Pilipets
Team members: Ana Marta M. Flores, Giacomo Flaim, Monika Skazedonig, Rita Sepúlveda, Serena Del Nero (alphabetical).
Project pitch slides
Final presentation slides
Key Findings
Introduction
Research Questions
Query Design & Visual Protocol
Methodology
Findings
Discussion
References
Key Findings
Telling a story of platform censorship critique through images poses specific methodological challenges related mostly to the contextual blurrings of hashtag-mediated visual content. Our understanding of context corresponds with the experiential, temporal and infrastructural (or grammatized) entanglements of user-platform interactions that translate into acts of visual circulation. When approaching the context of visual formations involved in Tumblr censorship critique with the aid of computer vision, both the content and the metadata connecting images require novel forms of understanding how images are situated in relation to one another.
The main findings of this project derive from the combination of nonhuman interventions into Tumblr subcultures and user resistance to these interventions through memes and other forms of vernacular participation (screenshots, GIFs, etc.).
By repurposing data-relational qualities of images (Google Vision API data, hashtags, timestamps, platform metrics) in the context of Tumblr porn ban, we were able to identify three overlapping dimensions engagement:
- Censorship hashtags (#censorhsip; #censored; #flagged) distributing screenshots of randomly flagged content rendered activities such as algorithmic flagging available for further analysis.
- Bot hashtags (#bots; #porn bots; #spam bots) helped us better understand the ephemeral dynamics of automated following through screenshots of porn bots ‘in action’.
- Memetic hashtags (#tumblr apocalypse; #tumblr purge; #female presenting nipples) varied both in their subcultural stance and their intensity of circulation, providing context-specific insights about the affective charge of Tumblr censorship critique.
Digging deeper into these relations requires both “thick visualisations” (Hochman & Manovich 2013) of aggregate data patterns and situational remapping of the same patterns attending to the ways in which Tumblr users reimagined the platform and its strategies of content distribution.
Especially when it comes to what Tumblr algorithms filtered incorrectly and what they overlooked, data-exploratory approaches focusing on the remediation of ‘prohibited’ content through visual means provide interesting entry points for methodological experimentation.
This project explores six months of image circulation following the event of porn ban on the social microblogging site Tumblr. In November 2018, after being temporary suspended from Apple’s App Store over a child pornography incident, Tumblr announced its decision to remove all sexually suggestive content from the platform by relying on machine-learning classification.
Why ‘censorship’ critique? The affective charge of Tumblr “purge”
On a platform that became known for its vibrant queer, body-positive and NSFW (not safe/suitable for work) hashtag publics (Byron 2019, Tiidenberg 2019, Paasonen, Jarrett & Light 2019), the new adult content policy provoked widespread criticism. Perceived by many as an economically motivated suppression of free speech rather than an act of protection from illegal content, the so-called Tumblr “purge” caused whole communities to move to other sites.
The subsequent fall in traffic, going from 521 million in December 2018 to just 370 in February 2019 (Statista 2020), was accompanied by users’ outrage about Tumblr’s miscarried attempts to eliminate porn spam and to fix the algorithm that was incorrectly flagging content. On top of it, the official language of new community guidelines prompted significant backlash during which the phrase “female-presenting nipples” went viral in a series of memes.
- How can we repurpose the networkedness of Tumblr content to map the unfolding of Tumblr censorship critique over time?
- How can we understand the particularities of engagement with “prohibited” content? Which story of Tumblr censorship critique can we tell by looking at these particularities through different forms of data visualisation/analysis?
- What can we learn about the affective charge of Tumblr purge critique from its memetic scenarios (across platforms)?
Query design and visual protocol
To detect relevant formations of Tumblr censorship critique in the wake of the porn ban, we first explored co-tag relations of #censorship (5673 posts) on Tumblr in the period between November 2018 (when Tumblr announced its new moderation policy) and August 2019 (when Verizon sold Tumblr to WordPress).*** After identifying the main patterns of association through Gephi network analysis, we made additional tag-based queries for #staff (15738 posts), #tumblr purge (10494 posts), #female presenting nipples (2622 posts), #tumblr apocalypse (2087 posts), #flagged (1462 posts), #censored (1349 posts), #yahoo (2268 posts), #verizon (1118 posts), #bots (938 posts), #pornbots (659 posts), #spam bots (503 posts). Using DMI Tumblr Tool, we opted for querying each term per month to be able to trace the transformation of associations over time. After merging the data in Google Sheets and removing the duplicates, this resulted in a dataset of 44,911 posts containing 15,158 images.
***A relevant hashtag that we were not able to query was #artists on tumblr. Although searchable on the platform, the hashtag returned no metadata when used as a query input into Tumblr Tool. This was also the case with “prohibited” content circulating through #NSFW-related hashtag-variations such as #N/S/F/W.
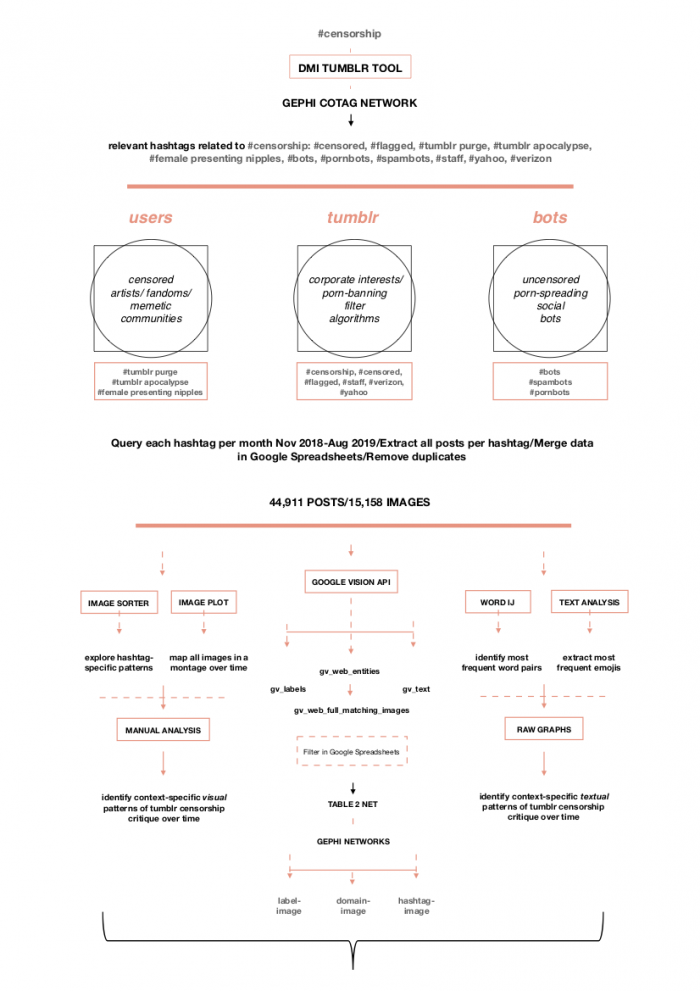
This query design reveals the relational nature of Tumblr porn ban, highlighting different forms of agency involved in the unfolding of platform censorship critique:
- Tumblr algorithms: #censorship, #censored, #flagged
- Tumblr staff: #staff, #yahoo, #verizon
- Porn bots: #bots, #porn bots, #spam bots
- Memetic communities: #female presenting nipples, #tumblr purge, #tumblr apocalypse
To capture these relations methodologically, we ran all 15,158 media URLs through Memespector python script, a tool processing visual content through Google Vision API. Drawing on the output of annotated data for each month containing 10 tabular (.csv) and 10 network (.gexf) files, we used the following affordances of Google Vision API annotation for further methodological experimentation:
- Detect labels: entities represented in an image, across a broad group of categories (helps arranging images into content-related clusters)
- Detect web entities: web references to an image (helps identifying memetic references)
- Detect adult content: the ‘likeliness’ of sexual content appearing in an image (“very unlikely”, “unlikely”, “possible”, “likely”)
- Detect sites of image circulation: URLs to Web images that fully match the image in the request (helps situating images in relation to external domains)
- Detect text: entire text strings, as well as individual words contained in an image (helps analysing screenshots)
Beyond exploring Tumblr-native data through different forms of visualisation and analysis (see visual research protocol and the methodology section) we also experimented with additional datasets (see descriptions below):
Additional datasets
Google Images: The dataset created by using the Google Image Search URL extractor contains a collection of the top 100 image URLs per month (using Google search specification after:yyyy-mm-dd before:yyyy-mm-dd) and image domain URLs returned for the neutral query “tumblr adult content”. It was used for (1). mapping the diversity of sources involved in the circulation of metacommentary on Tumblr porn ban and (2). exploring their visuality.
Instagram: The dataset created by using the DMI Instagram Scraper covers two months of #tumblrpurge and #femalepresentingnipples as well as other topical tags such as #fucktumblr, #tumblrisoverparty and #tumblrisdead (December 2018-January 2019). Although fragmented, it corresponds with the peak of tumblr censorship controversy. The visuality of hashtag publics shared by Instagram and Tumblr was then explored through combined hashtag-image networks.
To follow the reverberation of sentiment and critique in the context of Tumblr purge, we used different entry points into the relations of user-generated and platform distributed content by looking at its
- visual-narrative dimension (visual characteristics of the images, such as colours, text and image content)
- attentional dimension (grammatized dynamic qualities of the image, such as platform metrics/the count of ‘Tumblr notes’)
- sociotechnical dimension (contextual qualities of the image such as sites of circulation, time of posting, and hashtag-mediated publics)
By adapting mixed techniques of visual digital methods (for a recent discussion see Pearce et al. 2018; Niederer 2018; Niederer & Colombo 2019; Rogers 2019; Geboers & Van de Wiele 2020; Omena, Rabello & Mintz 2020), we approached the circulation of Tumblr censorship critique in three steps:
- Providing an over-time perspective
First, we mapped the unfolding of Tumblr censorship critique over time by creating three different visualisation types for Tumblr data: 1. Image plots (by plotting all images in accordance with the time of posting with ImagePlot and zooming in on the patterns of visual repetition in each of the months through Image Sorter). 2. Computer vision based image-label networks (by manually exploring, annotating and comparing the network file outputs of Memespector/Google Vision API). 3. Streamgraphs focusing on users’ textual metacommentary containing “prohibited” terms “NSFW” and “porn”. To create this visualisation, we extracted 10 most used word pairs from the post caption section of 7306 filtered Tumblr posts with WordIJ and visualised the results in accordance with the frequency of word pair repetition per month using RawGraphs.
Parallel to working with Tumblr data, we also created an image timeline (see also DMI19 summer school climate image spaces project report) based on the neutral Google Images query “tumblr adult content” using Google Spreadsheets =IMAGE(XY) formula, which provided us with a Google-recommended view of Tumblr porn ban. The particular ways in which Google recommends images/stories/sources for this neutral query in a specific period of time was then compared with the visuality of user-driven censorship critique on Tumblr.
- Zooming-in on relational particularities*
In the second step, we focused our attention on the peak of Tumblr censorship critique in December 2018, exploring the entanglements of different agencies and visual formats involved in remediation of prohibited content through different types of network analysis. To emphasize the shifts in relations of relevance unfolding in the respective contexts of user concern (related to algorithms, porn bots, censored female nipples, Tumblr’s commercial interests, etc.), we used platform rank data (the count of “notes” on Tumblr) to size the images when visualising them in networks. For this purpose, we built and explored different networks organising images in accordance with the combinations of selected hashtags and Google Vision API-detected text, labels, web entities, sites of circulation). This technique provided us with a relational understanding of content (screenshots, memes, GIFs, etc.) and its “derivative” data qualities (for a discussion of data derivatives in a different context see Amoore 2011) that resonated within various formations of Tumblr censorship critique.
- Capturing affect through memetic scenarios
In the third step, we explored the affective charge of Tumblr censorship critique through its memetic scenarios by visualising contextual variations of selected memes in grids (see e.g., Gaetano 2019). To identify relevant memes, we relied both on the manual annotation of image networks and image plots as well as on the filter function of Google Spreadsheets (by filtering the web entities column for specific memes such as “Surprised Pikachu” or “Confused Anime Guy”). In the course of analysis we also experimented with our additional datasets to compare Tumblr-native memetic scenarios with the visuality of Tumblr porn ban on other platforms (Instagram and YouTube). To work with YouTube content we used external image URLS extracted from Tumblr data. Instagram data was used for purposes of experimentation in building combined bi-partite networks of mixed platform data (Instagram and Tumblr) based on the shared hashtags “tumblr purge” and “female presenting nipples”.
*Note that networks created during the sprint (such as Google Vision API based networks) were further explored, transformed and interpreted through new visualisations in the course of preparing the research report.
When working with data coming from a platform that quickly and rather abruptly underwent significant content policy changes, different analytical possibilities arise from the ways in which ‘prohibited’ content was circulated. One of them is to look at the visual patterns of user-platform transactions over time.
- Providing an over-time perspective
Figure 2 presents a composite visualisation of four manually colour-coded image plot copies advanced by the visual affordances of the GIF (graphic interchange) format. The main visualisation was created using ImageMontage Plugin for Image Plot, resulting in a composite of 15, 158 images separated in temporal sequences of 10 months according with the time of posting.
The four colour-coded patterns of
- Tumblr announcing the new community guidelines
- Tumblr warning of adult content policy violations
- Tumblr informing users about new followers (porn spam) and
- Tumblr automatically flagging content
derive from Tumblr users’ screenshotting practices documenting automated notifications during the porn ban. This type of visualisation renders visible the immediate implementation of drastic content filtering measures mediated through users’ critique of algorithmic mistakes that Tumblr kept making. The intense screenshotting of notifications warning users of adult content policy violations (with the possibility of reviewing flagged content) in January 2019 (2) shortly after the new policy was announced (1) and immediately implemented (4) in December 2018 points towards Tumblr’s miscarried attempts at improving its algorithmic filtering strategies.
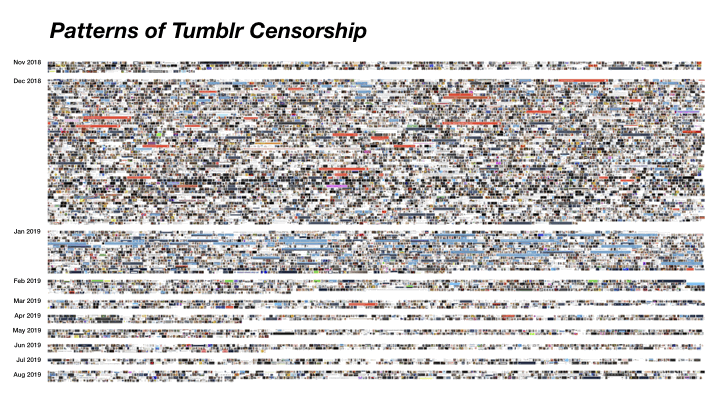
Figure 3 provides an overview of four notification types in relation to the frequency of their appearance in the Tumblr dataset per month. Captured through users’ screenshots, these notifications were filtered by using the text recognition module of Google Vision API, counted in Google Spreadsheets, and then visualised in an alluvial diagram using Raw Graphs. The four text queries that we used to filter the gv_text column in the spreadsheets were: “community guidelines” (1), “contain adult” (2), “started following” (3) and “was flagged” (4).
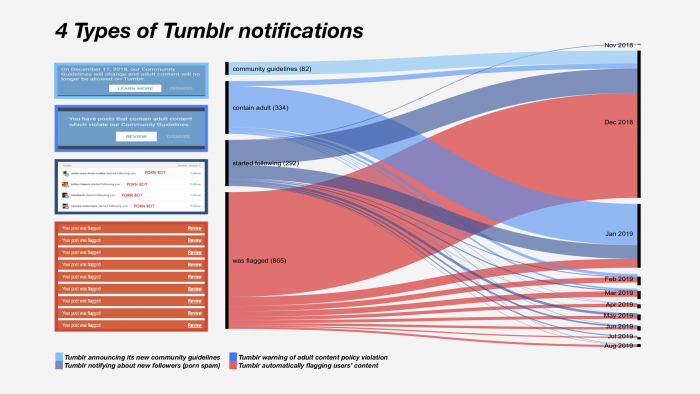
The distribution of “new followers”-screenshots capturing porn bot operations before, during and after the porn ban with two peaks of activity in December and January suggests a twist in users’ critique of Tumblr “censorship”. The main point of concern here was that while Tumblr was busy flagging porn-unrelated user content (4), porn bot followers successfully continued their operations without being noticed (3).
In our further explorations we focused on the relational particularities of Tumblr censorship critique inscribed in all other images in the plot that were not colour-coded. To do so, we explored the Memespector output of 10 bi-partite image-label networks in Gephi by looking at the formations of images structured through image content annotations provided by Google Vision API.
The strength of this approach lies in repurposing relational qualities of digital visual content while combining machine vision and network analysis with collective manual thick descriptions of image network clusters (see Mintz, Silva et al. 2019). These clusters are defined in accordance with the attribution of Google Vision labels to images that feature the same or similar entities (objects, faces, body parts, text, logos, animals, signs, etc.). Relying on the ForceAtlas 2 layout algorithm, the logic of network spatialisation in Gephi positions the nodes of the first type (labels) as network ‘coordinators’ that bring related images (the nodes of the second type) closer together while drawing unrelated images apart.
Figure 4 (click here to enlarge) presents a matrix of 10 image-label Gephi networks that were explored by comparing the visuality of images with the position of image labels and by manually annotating the clusters. The main purpose of this exercise was to identify persistent content formations unfolding through user critique of Tumblr porn ban and to explore the overlaps between the clusters at the core of the network.
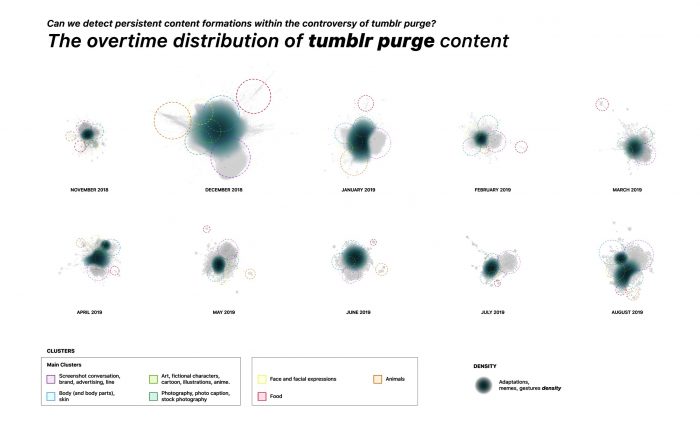
While comparing the distribution of shared labels presented in more detail in the figure 5 (click here to enlarge) with the actual appearance of plotted images, the main challenge that we encountered was dealing with the ambiguity of memetic content characterized by a high degree centrality or density of connections within the corresponding label clusters (see also the next section).
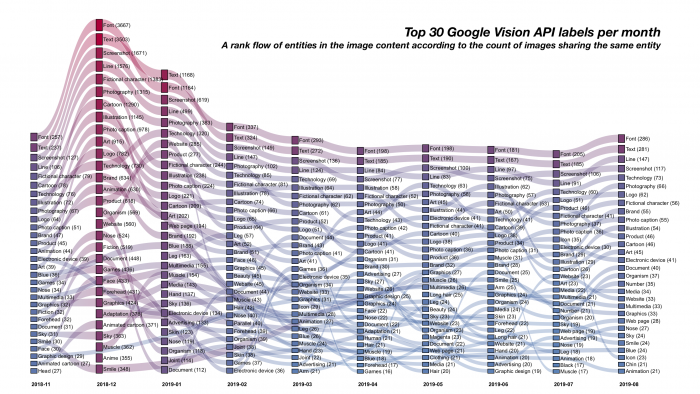
The persistent high density of connections around the labels such as “font”, “cartoon”, “photo caption”, “fictional character” and “screenshot” not only suggests a strong presence of memetic communities in the networks of Tumblr censorship critique. It also confronted us with major difficulties in identifying the shifts in user concern over time. Considering the dense mixture of visual subcultural references and variously attributed labels that were shared among them, we were able to locate memetic visuality around “fictional characters” and “photography” in each of the networks (figure 6). Text screenshots and screenshots of flagged body images were persistent as well: an indication of Tumblr’s ongoing issues with porn bots and the increasing amount of sarcastic visual comments responding to the enterprise of weeding out the “female-presenting nipples”.
Browsing through the image-clusters that were more homogeneous, we selected three months featuring the most typical imagery that helped us to understand the temporal dynamic of Tumblr porn ban. The image matrix in the figure 6 (click here to enlarge) presents five persistent visual clusters and one particular case for
- December 2018 (the black and white images announcing 24 hours until “the final day” before Tumblr’s new terms of service came into force on December 18th);
- May 2019 (screenshots of headlines when speculations came up that Pornhub was interested in purchasing Tumblr to ‘save’ the porn);
- and August 2019 (screenshots of headlines when Tumblr was sold to Automattic/Wordpress).
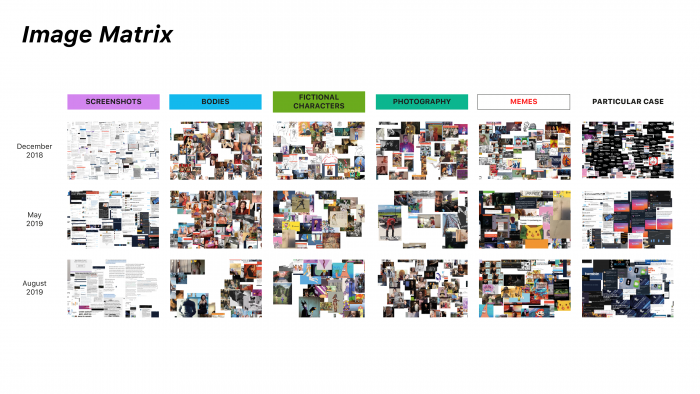
One of the most persistent visual clusters over time includes text screenshots that users were circulating to document unflagged porn bots “in action”. This element of critique came along with the problem of porn bot accounts which continued invading users’ blogs and comment sections after the porn ban without being noticed by Tumblr. The text content extracted from the screenshots using Google Vision API text detection module indicates the frequent use of links in combination with suggestive but not explicit visuality, often selfies. By distributing seemingly ‘innocent’ content containing invitations to “click here”, Tumblr porn bots quickly adapted to Tumblr’s new adult content policy, focusing more on the redistribution of user attention to external porn websites (for more on this attention economy see “Bots and the black market of social media engagement” project report by Omena, Chao, Pilipets et al. 2019).
This finding also corresponds with the textual analysis of post captions that were filtered in Google Spreadsheets for the use of prohibited terms “NSFW” and “porn” (figure 7). The distribution of fifteen most used word pairs over time shows not only the persistence of concerns with the porn bots and the subcultural struggle over “nsfw content” during the porn ban.
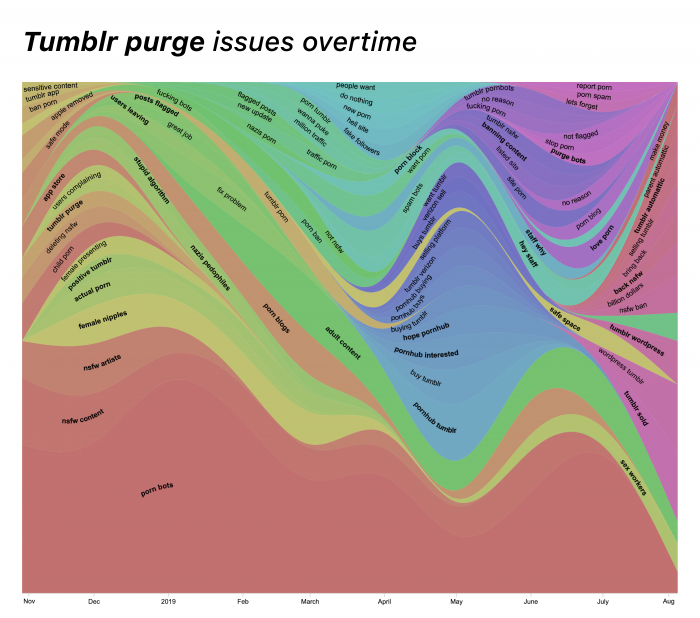
It also renders visible issue-specific variations of platform critique including outrage about the “stupid algorithm”, complaints about “nazis and pedophiles” infiltrating the site and the general distress of users leaving Tumblr for other platforms. Speculations about Pornhub’s interest in purchasing Tumblr in May 2019 and comments on the value loss of one billion dollars that Tumblr suffered after it was eventually sold to Automattic in August 2019 complete the story.
When comparing this situated hashtag publics’ view of Tumblr “censorship” critique (mediated through images and Tumblr post captions) with a Google images timeline retrieved from the neutral query “tumblr adult content”, two notable differences come to the fore:
The first is related to the use of the screenshots. Google-recommended view of “tumblr adult content” during the implementation of Tumblr’s new community guidelines is dominated by the screenshots of Tweets protesting against Tumblr porn ban. The use of Tumblr logo is also persistent. Images trending in the period between March and June 2019 feature graphs commenting on the drop in Tumblr traffic after NSFW communities were banned. Unlike on Tumblr itself, screenshots of flagged body images and porn bot interactions (figure 6) only rarely or do not at all appear in Google recommendations.
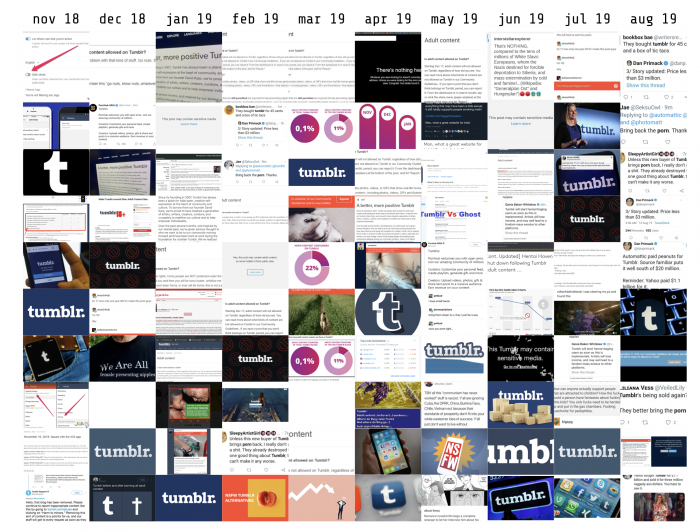
The second difference is related to the use of memetic content: Only in December 2018 Google features “female presenting nipples” memes combined with screenshots of the announcement of “a better more positive Tumblr”. This finding corresponds with the peak of creative user activity on Tumblr itself and indicates the situatedness of Google-recommended commentary in the journalistic trend of narrating issues through personal frames and catchy references.
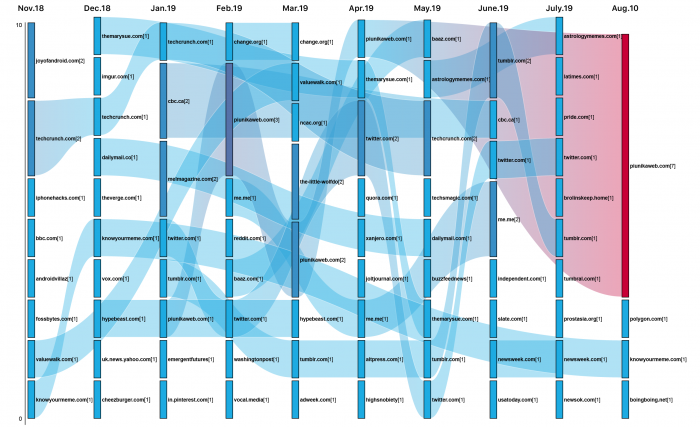
To show which Google recommended sources commenting on Tumblr porn ban were more persistent we complemented the image timeline with a timeline of image link domains: Beyond a range of well known online news and infotainment portals such as Techcrunch, The Verge, Dailymail and Buzzfeed News, a tech news website PiunikaWeb was most frequently recommended by Google. Notable about the site is that it used screenshots of popular Tweets (see e.g., top 5 images in August 2019) to update readers on the current status of Tumblr “adult content” ban.
- Zooming-in on relational particularities
Corresponding with the timeline of Tumblr NSFW content policy implementation, especially the spike in creative user activity in December 2018 provided interesting material for further exploration of the relational qualities (content labels, web entities, hashtag publics, post captions, sites of circulation) of flagged content and memes.
Labels, memes and flagged content
The image label network for December 2018 in figure 10 illustrates the challenges in dealing with a large amount of memetic content while relying on machine vision exclusively. It presents us with variations in the density of connections between Google Vision API labels and images that result in the irregular dispersion of fictional characters, photography, cartoons and fonts when they appear as plotted image clusters. However, groups of images within the clusters attributed to the same colours- and text-labels are more readable (note the close proximity of black and white images announcing 24 hours until “the final day” of Tumblr within an otherwise highly dispersed cluster of memes).
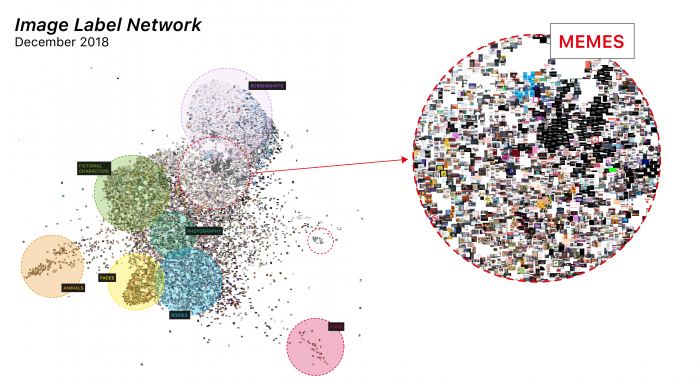
The consequence of this logic is that formations around well recognizable visual patterns (e.g., faces) tend to be more homogeneous than formations around creative content with multiple references or ambiguous entities contained in the image (see figure 11).
As presented in the figures 10 and 11, the degree of ambiguity in image content translates into the logic of spatialisation of the image network in accordance with the distribution of content between the center and the periphery. The more ambiguous the content, the more dense the degree centrality in the mid-term of the network and the more dispersed its formations. The more distinct the content (faces, food, animals), the more concentrated its formations at the periphery, thus suggesting a specific correspondence between the weight and the meaning of connections between the images in a network (to learn more about the logic of network spatialisation, see “cross platform digital networks” project report by Omena et al. 2020).
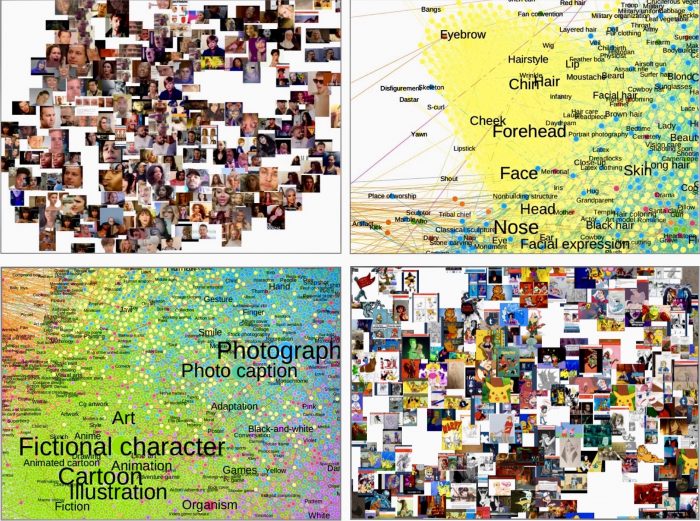
An interesting finding that comes from the interpretation of these connections is the dispersed position of the image nodes that represent the same (or visually similar) image but are defined by multiple groups of labels at the same time. Three different groups of Google Vision API labels describing the content of a “Surprised Pikachu” meme provide an illustrative example (note the distribution of the meme across the “fictional character”-“adaptation” clusters in the figure 11 (bottom right) and compare three different ways to label Pikachu in the figure 12:
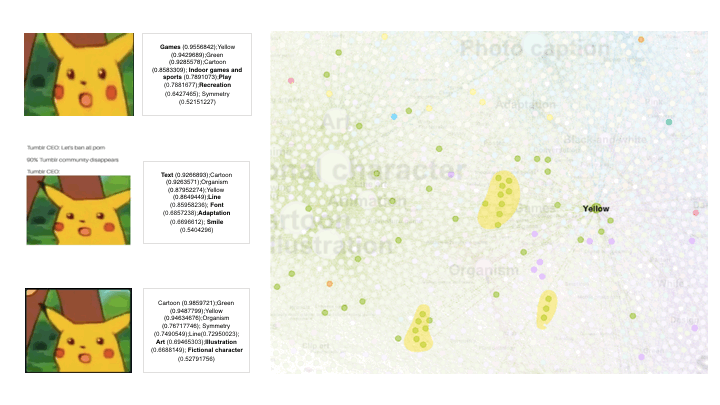
While unambiguous qualities of the image such as colors are shared in all three label groups, more specific label attributes that Google Vision API recognises in the same image – such as “games”, “adaptation”, and “fictional character” – define the dispersed position of the meme in the network. The attribution of the label “organism” characterising two of the three Pikachu variations in combination with “fictional character” and “adaptation” labels, points to the challenges of automated image recognition when it comes to the understanding of context.
However, when rebuilding the December 2018 image-label network in accordance with its singular aspects, for instance, by zooming in on the screenshot formations attributed to specific hashtags, Google Vision API labels have proven themselves very useful in recognising the visual patterns within related image clusters. Before the data sprint such a network for #flagged was created to see which kind of content was mistakenly flagged by Tumblr filtering algorithms in December 2018.
In the figure 13, a sample of #flagged contributions featuring fictional characters, cat photos, erotic selfies, text-based memes, and lingerie models captures the challenges of implementing untrained image recognition algorithms when it comes to the understanding of context (only 16 of 331 images that Tumblr identified as porn were labeled by Google as “likely adult” in the clusters “body” and “fictional character”).
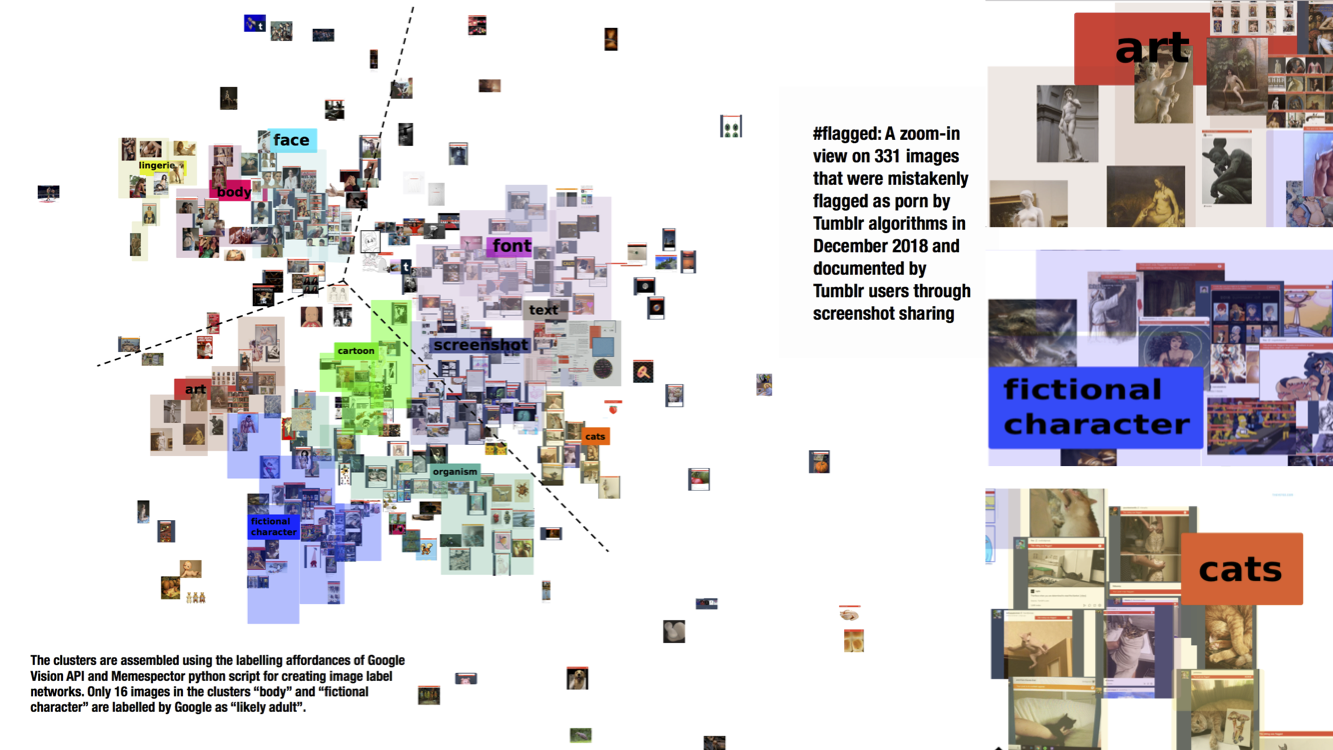
Superimposed on most of the images, Tumblr’s red flag message reading “Your post was flagged” points to unjust platform interventions in a range of user actions. This gives us an idea about the entanglements of Tumblr censorship critique with two forms of agency: Tumblr porn filters that randomly removed all kinds of content from public view and Tumblr users who were screenshotting and reposting this content as a form of documenting the inadequacy of the porn banning algorithm.
Web entities and sites of circulation
To find an alternative way of dealing with ambiguous image data through network analysis, we used Google Vision API’s web entities instead of labels to re-organise images in accordance with their web references. To do so, we built a test network for 500 most reblogged, liked and commented #female presenting nipples images that were filtered in Google Spreadsheets by the count of notes. Unlike labels that are attributed to the objects and object properties in a descriptive way, web entities also provide information about the cultural references and the format of the images as well as the platforms that host them.
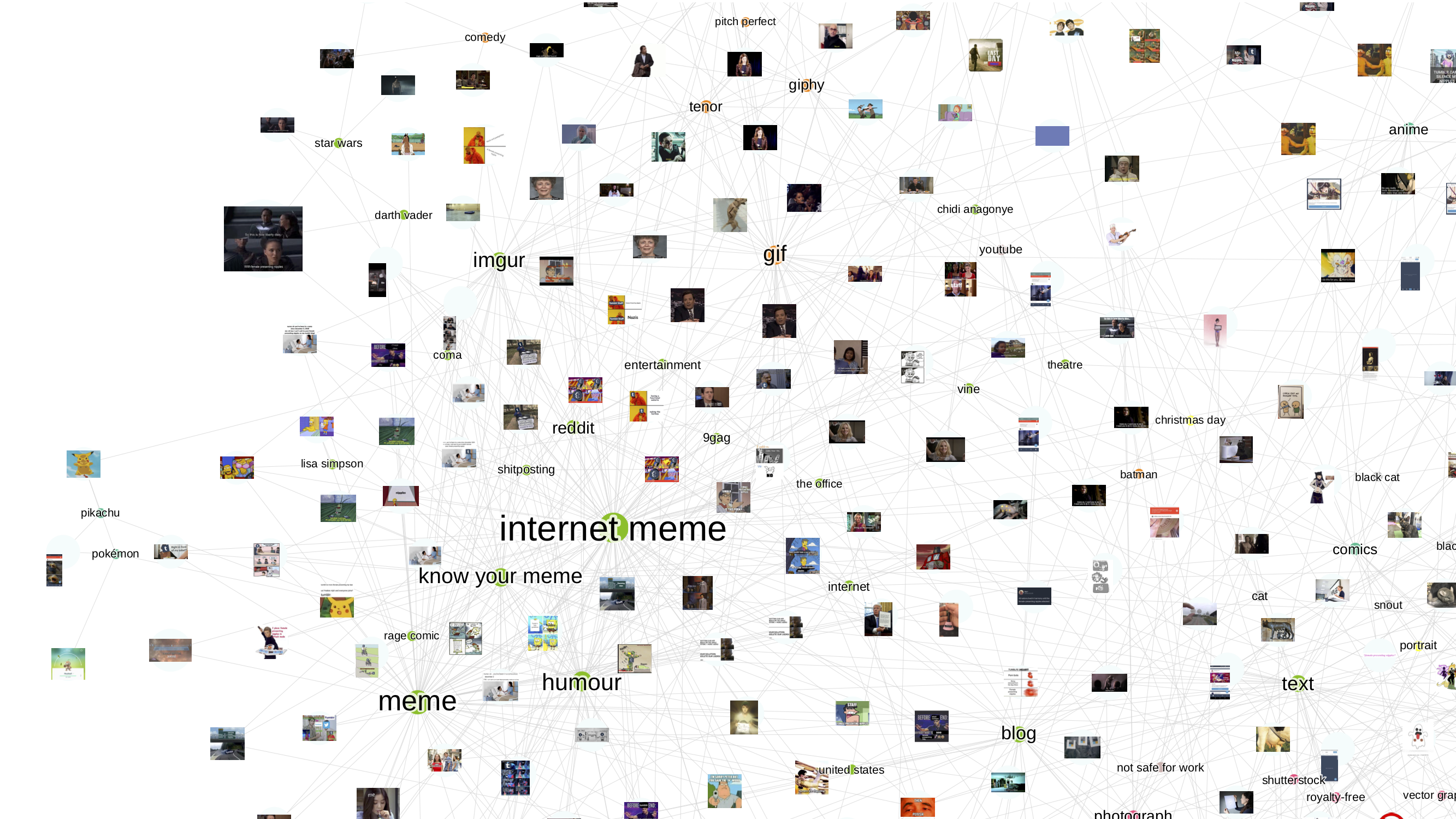
Figure 14 zooms in on the biggest cluster of the network – internet memes, assembling the images in accordance with their format (meme, GIF, text, stock photography), cultural references (Pikachu, Rage comic, Star Wars, Pitch Perfect, etc.), stance (shitposting, joke) and the corresponding platforms (Know Your Meme, Imgur, Giphy). The size of the images represents the resonance of each image on Tumblr, highlighting the popular Star Wars parody of Senator Amidala’s line “So this is how liberty dies…”, which gained 39,184 notes (Tumblr reblogs + likes + comments) by the end of January 2019 and was featured on KnowYourMeme as part of the “female presenting nipples” series on Tumblr.
The appearance of popular image repositories in the network suggests that Google relies on these platforms to train their image recognition models. The relation of these repositories to the sites of image circulation needs to be further explored by combining web entities with the information identified through Google Vision recognition of “full matching images”.
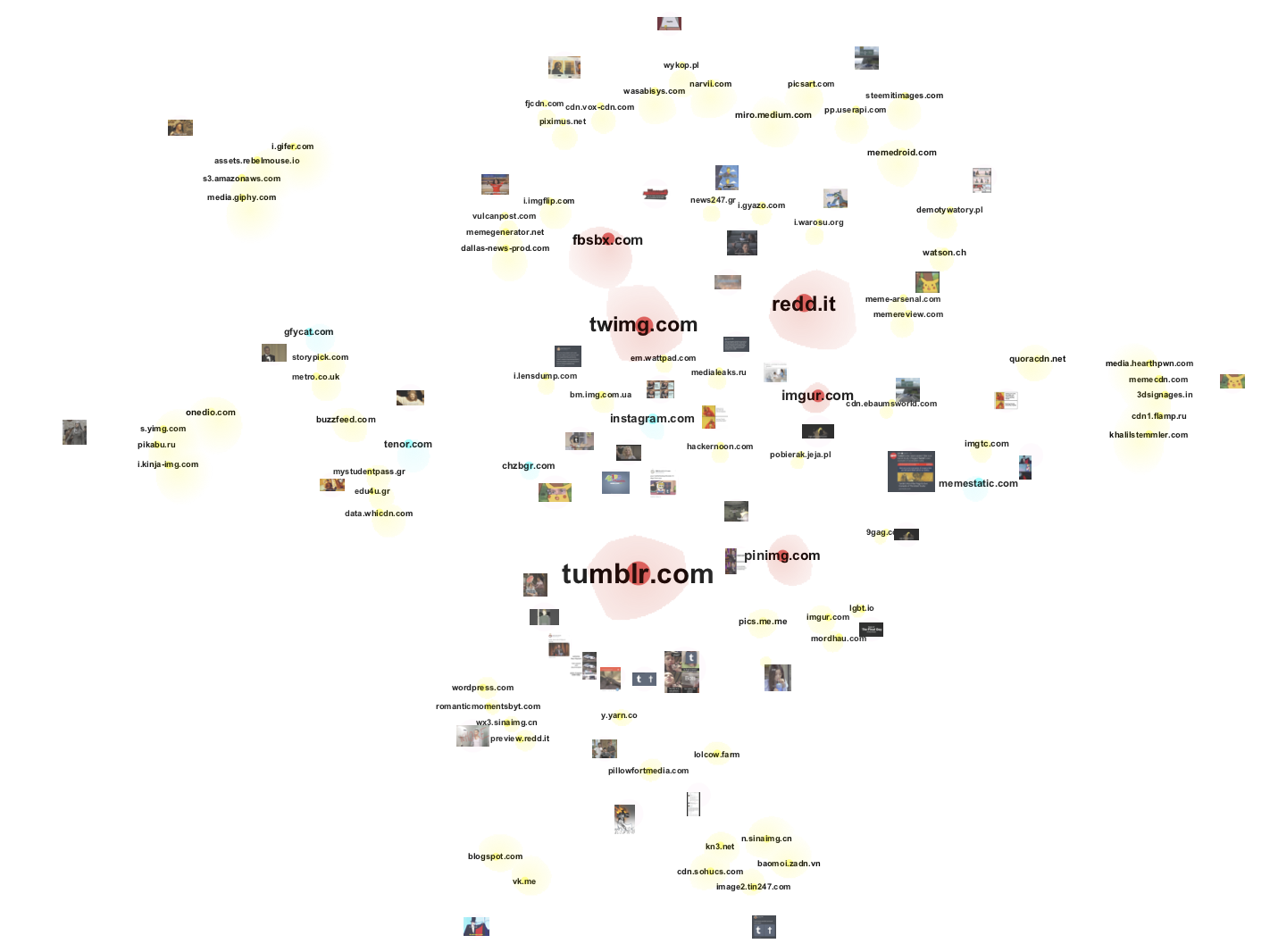
After the sprint, we tested 50 most popular images that Google identified as memes by creating a small image-domain network to see which platforms were featured as the hosts of images fully matching the appearance of the images in the request (Figure 15). As expected, especially when dealing with popular memes, this might not be the best method: In most cases, the domains returned by Google (mainstream platforms and meme repositories such as imgur.com) feature the same image but not the same meme (text captions contained in full matching images are usually random).
However, in the case of more specific memes created in the context of Tumblr porn ban (like the burning Tumblr logo), Google returned domains with the images that were indeed fully matching. Pillowfort – a platform allowing sexual content and NSFW art was among these domains, pointing to the sites of circulation that presented themselves as Tumblr alternatives during the porn ban. Although potentially interesting in terms of the analysis of circulation, these findings require further exploration and clarification.
Memetic hashtags: #tumblr purge and #female presenting nipples
The exploration of three different types of hashtag-image networks allowed us to trace evolving impressions of Tumblr porn ban as imagined and documented by users. What came out of the analysis of all networks is telling of the challenges that result from algorithmic interventions into platform communities.
First, skin-coloured content containing body shapes can easily become a source of ‘false positives’. And this is what we learned from all the nipples that were photoshopped upon all kinds of images on Tumblr in the context of #female presenting nipples hashtag publics. Responding to censored art that had been mistakenly flagged as porn, the visuality of this hashtag was characterised by typical practices of remediation through object-based memes and art appropriations. In the figure 16 network fragments focusing on the visual elements of #female presenting nipples illustrate various techniques of vernacular creativity that were used to ‘dare’ Tumblr’s content filtering algorithm (note the hashtag cluster coming from a Tumblr account dedicated to creating and reposting the most absurd #female presenting nipples objects).
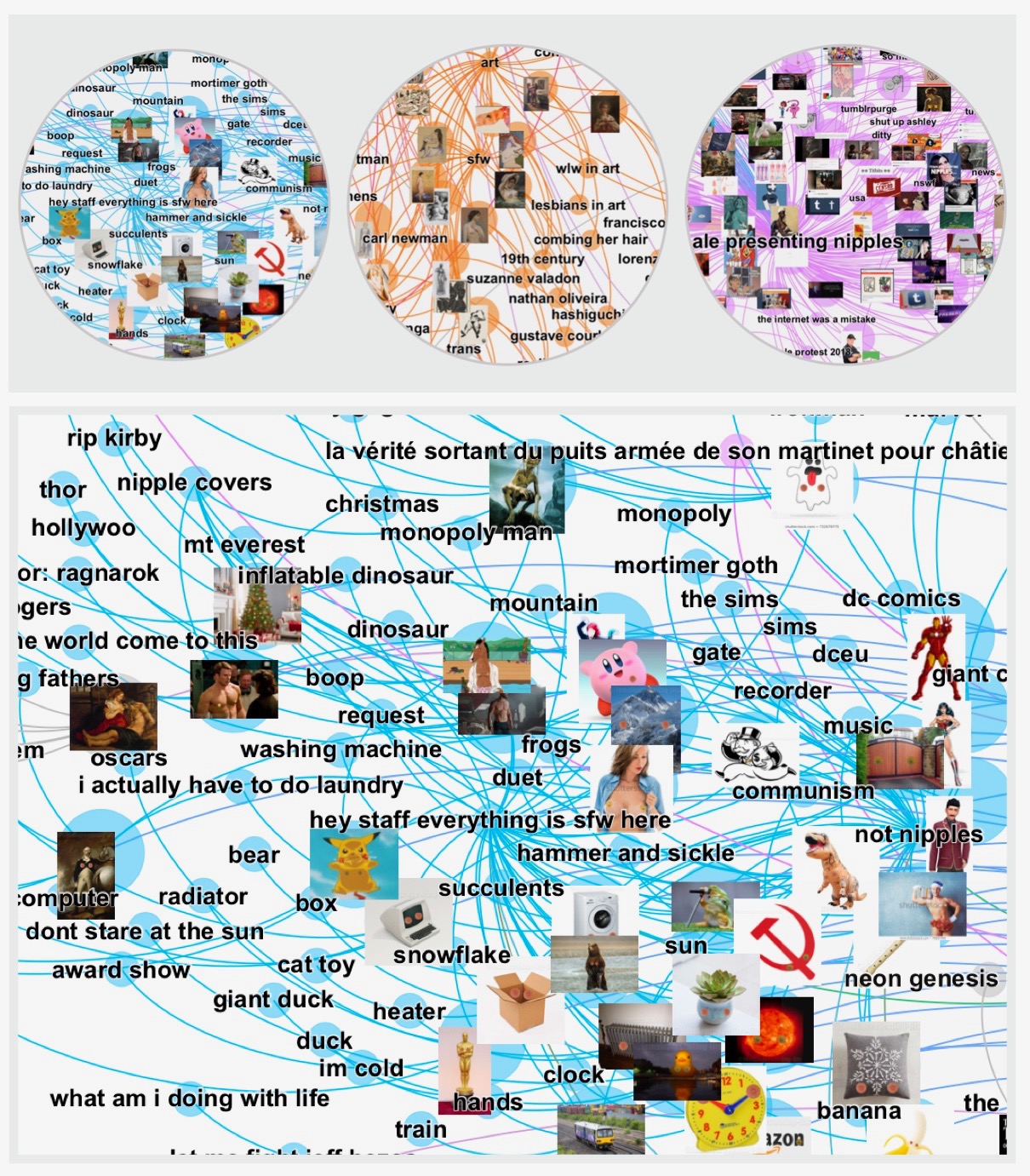
The second network focuses on the intermingling of different forms of visual expression through hashtags in relation to the attentional dynamics of content distribution. An important methodological note here is that to provide this relational view (see figure 17), we worked with a combined hashtag-image network assembling 25 unique posts for each of the 12 selected hashtags (see query design) containing the terms “NSFW” and “porn” in the image captions. Instead of focusing on the top shared posts, images and co-tags attached to separate hashtag queries, this technique enabled us to explore how user critique of Tumblr porn ban registered in variously weighted hashtag-image combinations.
Popular contextual adaptations of #tumblr purge, #tumblr staff and #female presenting nipples in the figure 17 played an important part in understanding the experiential qualities of Tumblr use during the porn ban. Interlinked through co-tags such as #protest,#december 17th, #tumblr ban and #rip Tumblr, especially reaction GIFs and GIFs mimicking crisis situations were used as an alternative means of affective alignment. In this context, Surprised Pikachu became the main memetic face of Tumblr purge thanks to a highly engaging GIF variation that received 57680 Tumblr notes in December 2018 (see also figure 20).
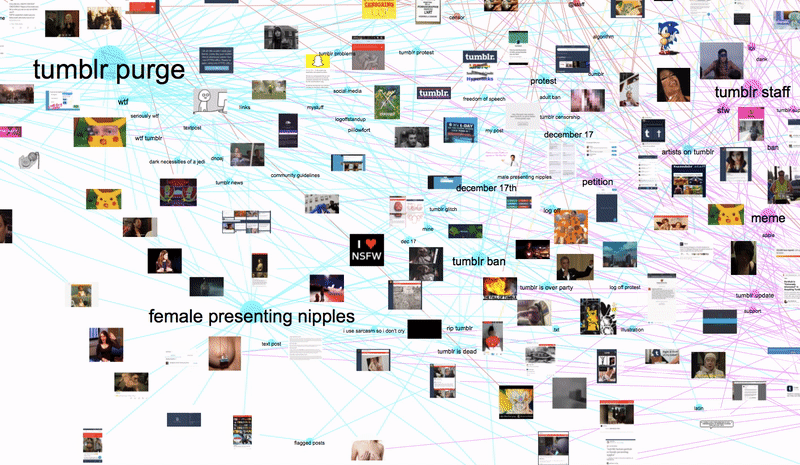
The third type of hashtag image-network was built to compare the visuality of #tumblr purge and #female presenting nipples hashtags on Instagram and Tumblr. To do so, we assembled a dataset of 400 images through two Tumblr (#tumblr purge; #female presenting nipples) and two Instagram (#tumblrpurge; #femalepresentingnipples) hashtag queries, creating a merged network file of hashtags and images coming from two different platforms.
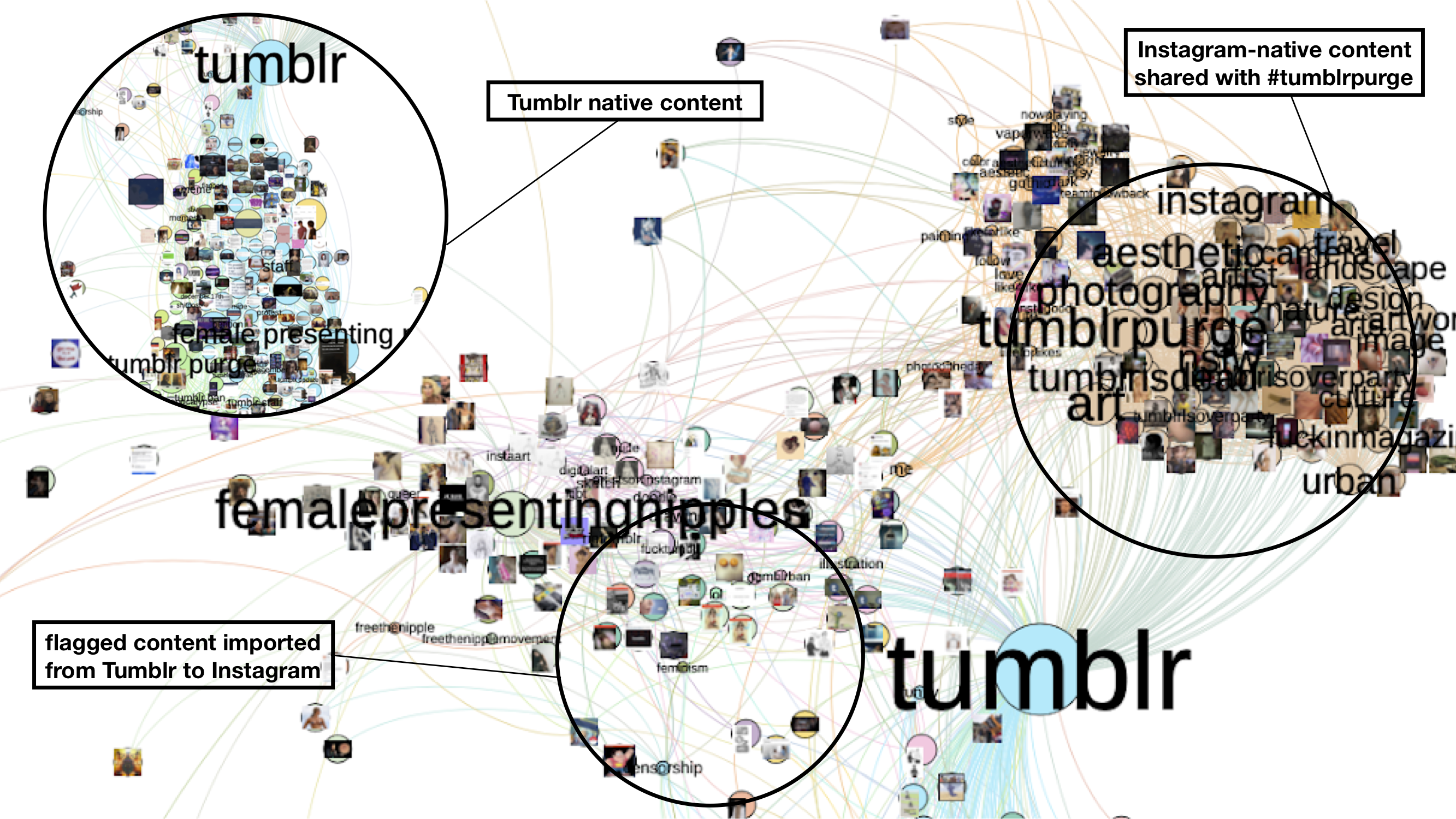
The network fragments in figure 18 highlight the ambiguity of hashtags as mediators of issue publics and the specificity of platforms as well as their respective cultures of use. For instance, the use of #tumblrpurge on Instagram was not issue-related, but connected to an influential Instagram account dedicated to urban trends and travel aesthetic imagery instead. The only shared #tumblr purge co-tag between Instagram and Tumblr was #tumblr.
Instagram #femalepresentingnipples imagery was indeed used in the context of Tumblr censorship critique. Here, again, screenshots of flagged content played an important role as a means of importing Tumblr-native content to Instagram. In addition to the main co-tag #tumblr, Tumblr and Instagram #female presenting nipples images also shared the co-tags #censorship and #feminism.
- Capturing affect through memetic scenarios
Considering that the intended uses and platform-specific formations of engaging content vary greatly,we conceptualise memes as data-intensive relational objects that differ not only in their stance but also in their intensity of circulation. This intensity is what we describe in terms of memefication of Tumblr censorship critique, while taking into account the capacity of internet memes to affect new acts of appropriation and sharing both within and across platforms (see also Figure 15).
Drawing on the results of manual thick descriptions of network clusters, we were able to identify and visualise five main scenarios of memefication by using Google Vision “web references” to filter the whole December 2018 dataset for “Surprised Pikachu”, “Confused Anime Guy”, “Drakeposting”, “Distracted Boyfriend” and “Simpsons”. Figure 19 shows top 10 most liked and reblogged adaptations per meme. For each memetic scenario, the results have been ranked, ordered, annotated and color coded in Google Spreadsheets.
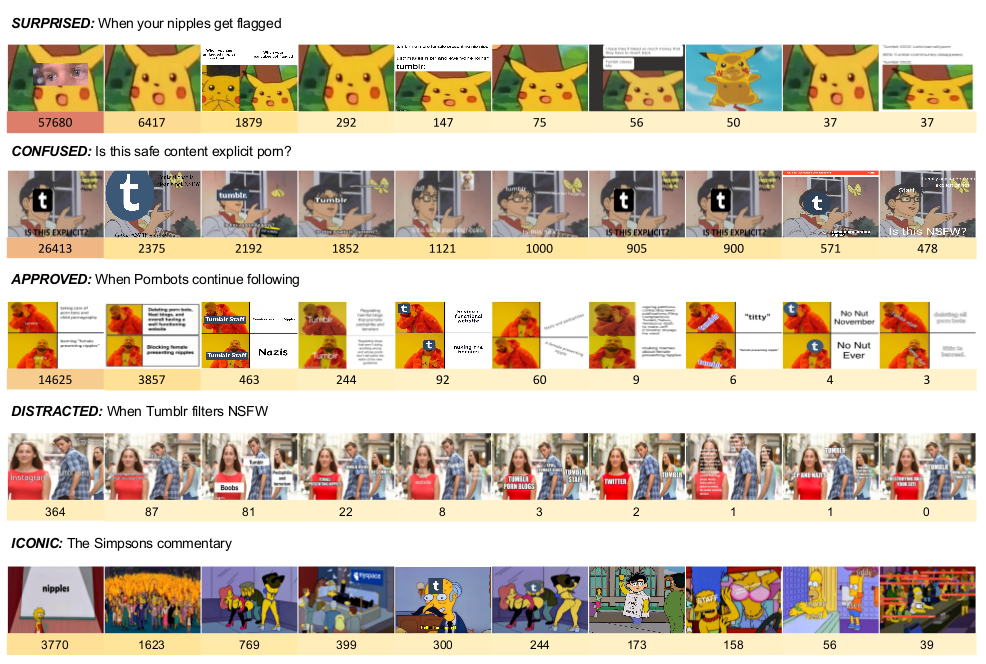
This analytical technique captures recurring themes and variations of stance in memetic scenarios of user alignment, corresponding with the following dimensions of Tumblr censorship critique:
- ‘distracted’ Tumblr staff (impersonated by ‘Drake approves/disapproves’ and “distracted boyfriend” memes suggesting that Tumblr preferes porn bots, Nazi blogs and child pornography to “female presenting nipples” and NSFW artists)
- ‘confused’ porn banning algorithms (impersonated by “confused anime guy” – initially Katori Yutaro, a popular android anime character who keeps confusing one thing for another thing)
- ‘surprised’ user communities and NSFW artists (impersonated by variations of Pikachu wondering as to why their “nipples” get flagged while porn bots keep spamming)
Unlike screenshots documenting ‘unjust’ interventions of porn bots and filter algorithms into Tumblr communities, pop culture references circulating through memes and iconic adaptations of “The Simpsons” give us an idea about the affective charge of Tumblr censorship critique through a lens of sarcastic metacommentary (Phillips & Milner 2017). Accumulating thousands of Tumblr reblogs and likes during the peak of user resistance in December 2018, memetic hashtag publics strangely intensified engagement on a platform that was about to lose more than 30% of its traffic.
This experiential-expressive ambiguity of Tumblr porn ban is also what we encountered while analysing emojis. Figure 20 presents a hierarchy of emoji gestures, symbols, objects and faces that were extracted from the image captions using TextAnalysis, manually coded in Google spreadsheets and visualised in a RawGraphs’ treemap.
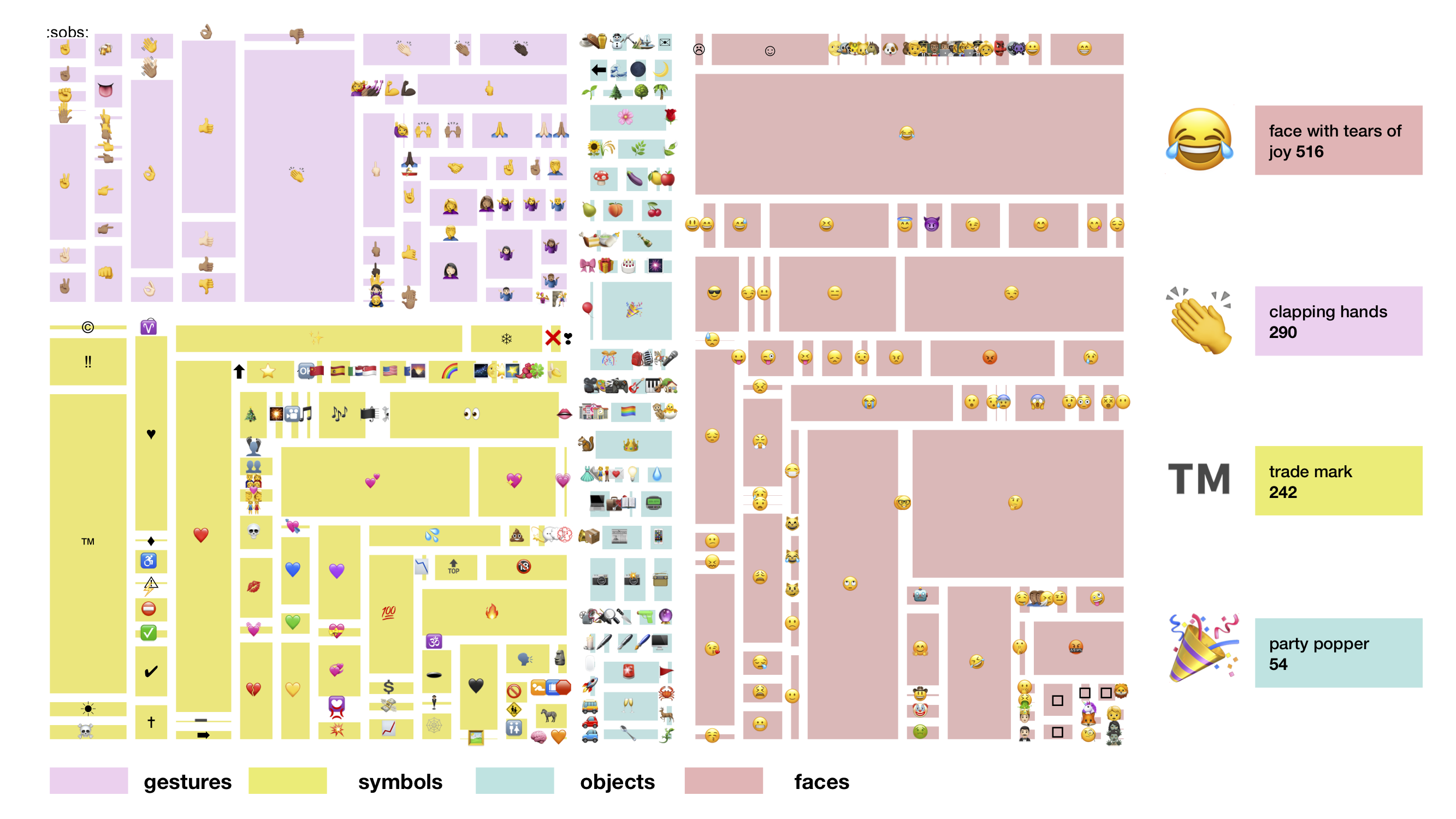
The most used emojis – “face with tears of joy” and “clapping hands” – were identified in the image captions of the screenshots documenting Tumblr notifications of new porn bot followers. Used as sarcastic markers of Tumblr’s miscarried attempts to ban porn, these emojis also frequently appeared together with “party poppers” in the context of Tumblr “log off” protests in December 2018. The ™ emojis were used to point out the weirdness of this all as “typical” for Tumblr through comments such as “literally less than 2 days after the Purge™️ and there’s been EIGHT” (porn bot followers) or “Tumblr Madness™️”.
Another important memetic particularity was shaped by YouTube-native metacommentary. Circulating on Tumblr during the first three months of the porn ban, especially three contributions gained traction (figure 21):
- The appropriation of Titanic’s “Gentlemen, it has been a privilege playing with you tonight” after the porn ban was announced and NSFW artists started leaving Tumblr (55, 243 notes on Tumblr, deleted on YouTube due to copyright reasons, continues to circulate in reaction videos).
- The viral video parody by Prozd making Tumblr appear as a platform who desperately wanted to be out of business (2,242,268 Youtube views)
- The viral video parody by CollegeHumor “Tumblr CEO: No more porn” (4,314,293 YouTube views) mocking the idea of the new ‘safe’ Tumblr
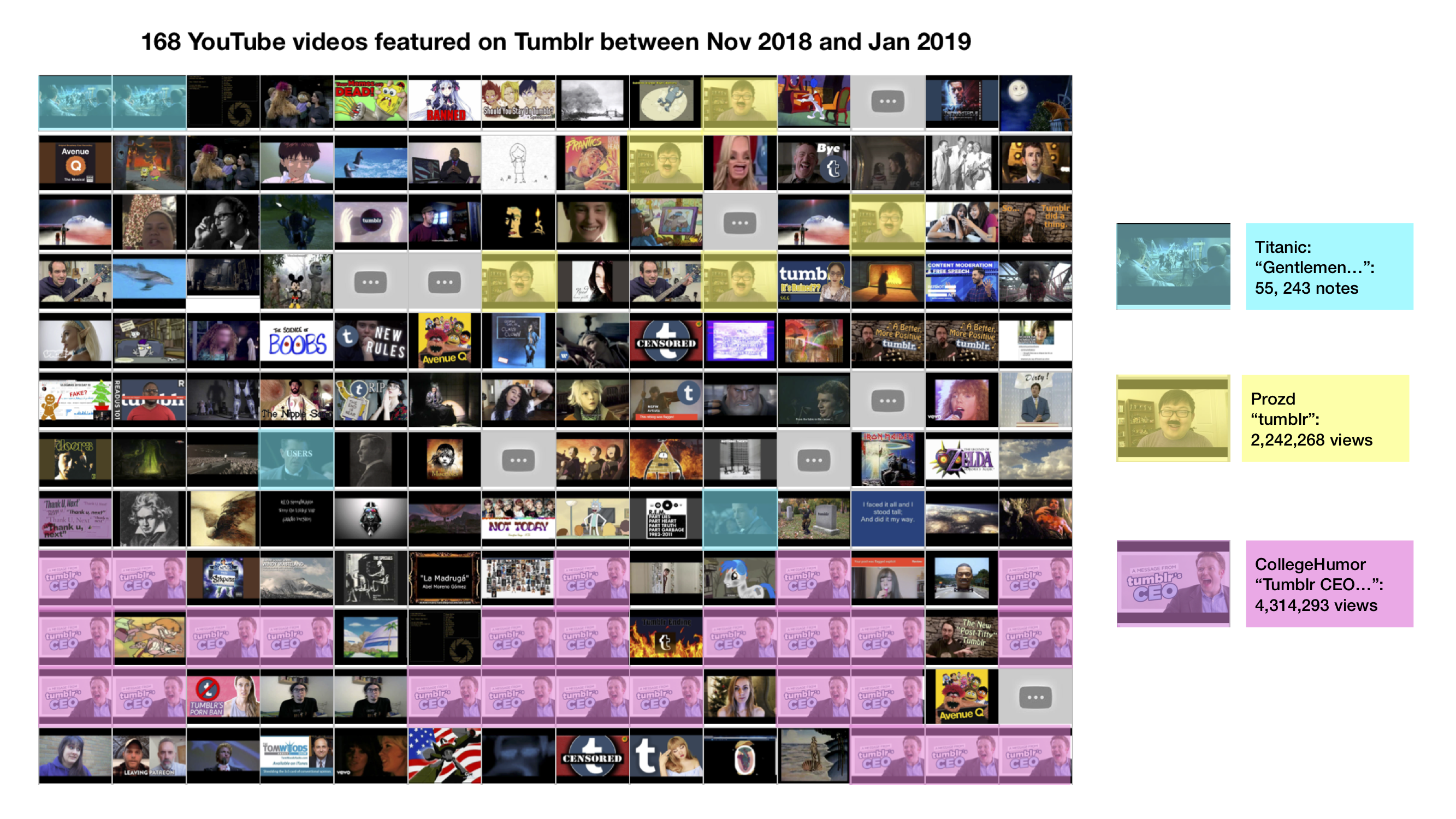
The hashtags that were used to circulate these videos were related to #tumblr purge hashtag publics and #december 17th log off protests, corresponding with the context of memefication of Tumblr censorship critique. Our understanding of this context derives from the capacity of memetic content to provoke engagement, which requires accounting for the intermeshing of the attentional, narrative and sociotechnical dimensions of action in online exchanges. Seen methodologically, (cross-platform) variations in memetic scenarios of Tumblr censorship critique invite to explore networked qualities of platform visual vernaculars alongside their manifestation as objects of circulation and mediators of affect (Paasonen, Hillis, Petit 2015; Niederer 2018; Pilipets 2019).
This project has explored methodological possibilities that arise from the dynamics of content circulation on a platform undergoing abrupt content policy changes. By repurposing the affordances of Google Vision API data in combination with networked qualities of user critique in the wake of Tumblr porn ban, we specifically focused on the engaging potential of internet memes and other visual platform vernaculars such as screenshots.
Observed in combination with hashtag-mediated practices of image-sharing, these data-relational qualities provide us with a situated view of Tumblr-specific “grammars of action” (Agre 1994; Gerlitz 2016) during and after the porn ban (e.g., flagging). The partiality of this view deriving from the restrictions in use of the new “more positive” Tumblr produced questions pertaining to the ways in which situated or fragmented visual data can be understood in its “derivative” (Amoore 2011) potential:
The study of networked images usually derives from an amalgam of contextually dispersed data that always already embed specific ways of thinking images in relation to other images. The methodological praxis of changing the relation between data elements within and across different datasets (by relying on different combinations of images and metadata), supports the situated work of interpretation. Especially when dealing with multiple contextual adaptations of memes, this derivative potential of platform data can be repurposed as a narrative format through composite visualisations drawing together data objects into new visual artifacts (Niederer and Colombo 2019).
Linking images in new formations – based on the use of hashtags and image captions in combination with Google Vision API labels and web entities – produced valuable entry points into the exploration of how networked screenshotting practices and their different scenarios of use were turned into memetic material. For now, hashtag-mediated formations of image sharing and Google Vision API web entities proved to be the most productive sites of methodological experimentation, especially in terms of dealing with the memetic components of intertextuality, humour and imitation. Further investigations of memes through Google Vision API afforded concepts such as full matching images can valuably add to accessing the dynamics of memetic circulation and cross-platform appropriation.
Composite visualisations that were designed to make sense of these relations were either repurposed as a narrative format or as situated thick descriptions of a particular aspect of Tumblr censorship critique: For instance, by making visible again that which has been made invisible (random subcultural content) or ignored (porn bots) by Tumblr filters, screenshots became a central means of user resistance, significantly contributing to its memefication.
Taken together, these different visual vernaculars tell us a story of platform interventions in user cultures. However, an important limitation of this story is that it obviously does not reveal the whole picture. What it does though is letting visual inscriptions of user-platform interactions create their own narrative. Among the main advantages of this approach is its focus on the shifts in relations of critique, concern, humor and care under conditions of deplatforming.
Agre, PE (1994) Surveillance and Capture. Two Models of Privacy. Information and Society 10(2): 101-127.
Amoore, L (2011) Data Derivatives: On the Emergence of a Security Risk Calculus for Our Times. Theory, Culture & Society 28(6): 24-43.
Byron, P (2019) ‘How could you write your name below that?’ The queer life and death of Tumblr. Porn Studies 6(3): 336-349.
De Gaetano, C (2019) Looking for Climate Change Solutions on Instagram. The Medium. Available at: https://medium.com/digitalsocietyschool/looking-for-climate-change-solutions-on-instagram-9b16ed7b0b5ahttps://medium.com/digitalsocietyschool/looking-for-climate-change-solutions-on-instagram-9b16ed7b0b5a
Geboers, M, Van De Wiele, CD (2020) Machine Vision and Social Media Images: Why Hashtags Matter. Social Media + Society 6(2): 1-15.
Gerlitz, C (2016) What Counts? Reflections on the Multivalence of Social Media Data. Digital Culture & Society 2: 19-38.
Gerlitz, C, Helmond, A (2013) The like economy: Social Buttons and the data-intensive web. New Media and Society 15(8): 1348-1365.
Lovink, G, Tuters M (2018) Rude Awakening: Memes as Dialectical Images. Non.Copyriot. Available at: https://non.copyriot.com/rude-awakening-memes-as-dialectical-images/.
Mintz, A, Silva, (2019) Interrogating Vision APIs. SMART Data Sprint Research Report. Available at: https://metodosdigitais.fcsh.unl.pt/smart-2019/project-reports/interrogating-vision-apis/.
Niederer, S (2018) Networked Images: Visual Methodologies for the digital age. Amsterdam: Hogeschool van Amsterdam.
Niederer, S, Colombo G (2019) Visual Methodologies for Networked Images: Designing Visualizations for Collaborative Research, Cross-Platform Analysis, and Public Participation. Disena (14): 40-67.
Omena, JJ, Chao, J, Pilipet, E, Kollanyi, B., Zilli, B., Flaim, G., Sívori, H., van Ruiven, Kim, Rademakers, L., Li, M. & Del Nero, S. (2019) Bots and the Black Market of Social Media Engagement. Digital Methods Initiative Summer School Wiki. University of Amsterdam. Available at: https://wiki.digitalmethods.net/Dmi/SummerSchool2019Botsandtheblackmarket
Omena, JJ, Rabello, ET, Mintz AG (2020) Digital Methods for Hashtag Engagement Research. Social Media + Society: 1-18.
Paasonen, S, Hillis K, Petit, M (2015) Introduction. Networks of transmission: Intensity, Sensation, Value. In: Hillis, K, Paasonen, S, Petit, M (ed.) Networked Affect. Cambridge MA: MIT Press, pp. 1-26.
Paasonen, S, Light, B, Jarrett, K (2019) The Dick Pic: Harrassement, Curation, and Desire. Social Media + Society 5(2): 1-10.
Pearce W, Özkula SM, Greene A, et al. (2018) Visual cross-platform analysis: digital methods to research social media images. Information, Communication & Society: 1-20.
Pilipets, E (2019) Contagion Images: Faciality, Viral Affect and the Logic of the Grab on Tumblr. In: Schober-de Graaf, A (ed.) Popularisation and Populism through the Visual Arts: Attraction Images. London: Routledge, pp. 161-180.
Phillips, W, Milner, RM (2017) The Ambivalent Internet: Mischief, Oddity, and Antagonism Online. Cambridge: Polity Press.
Rogers, R (2019) Doing Digital Methods. Massachusetts: MIT Press.
Rose, G (2016) Visual Methodologies. An Introduction to Researching with Visual Materials. London: Sage.
Rose, G, Willis, A (2018) Seeing the smart city on Twitter. Colour and the affective territories of becoming smart. Environment and Planning D: Society and Space 37: 411-427.
Tiidenberg, K (2019) Playground in memoriam: missing the pleasures of NSFW tumblr. Porn Studies 6(3): 363-371.